September 15, 2023 | Science Park Amsterdam
PAST EVENT. RECORDINGS AVAILABLE NOW!
TRANSFORMERS AT WORK
4th edition
After a very fast rise, Transformers have become the work horse of modern AI. From edge devices to the largest language models in the cloud, computer vision, robotics, reinforcement learning, they are everywhere. Even modern hardware has become optimized to the specifics of this Deep Learning architecture.
In the 4th edition of our workshop you will learn about the progress in Transformers from world-renowned researchers pushing the boundaries in their respective subfields such as Neural Information Retrieval, Conversation Agents, Large Language Models, and Multimodality. In contrast to earlier editions, the focus will be more on the shift towards real world applications of AI.
After the workshop food, drinks + live music (Roda da Holanda) create a unique opportunity to meet and have fun with fellow AI R&D folks from Industry and Academia at the beautiful Startup Village Amsterdam!
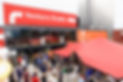
Meet our speakers:
New edition, all the more value
LEARNING
We are proud to welcome researchers and leaders from industry and academia. Our speakers will show you the latest developments & most promising advances in the field. The starring role is of course reserved for Transformer models.
DISCOVERY
Advances in NLP and Search are changing the way AI systems can process unstructured data, which makes understanding of the state of the art and its implications for applications critical to getting ahead in virtually any industry.
NETWORKING
Make valuable connections and meet experts and peers not only in the AI industry, but also in all others touched by tech. "If you want to go somewhere, it is best to find someone who has already been there".
FUN
To celebrate the amazing progress in our field and kickstart the academic year with some fun, we can't conclude the evening without some entertainment. Drinks, food, & live music included, good company guaranteed!
What you can expect:
Time schedule:
12:00-13:00 Arrival and registration
13:00 Opening: Jakub Zavrel
13:30 - 14:45 Suzan Verberne, Nils Reimers, Rodrigo Nogueira
14:45 - 15:15 Coffee Break
15:15 - 16:30 Raza Habib, Madelon Hulsebos, Douwe Kiela
16:30 - 16:45 Break
16:45 - 17:30 Corina Gurau, Konstantinos Bousmalis
17:30 - 18:00 Panel "Transformers at Work"
18:00 Drinks, Food & Networking
19:30 Live music: Roda da Holanda
22:00 End
Find the full list of titles and abstracts below.